Generative AI: What It Is and How It Works
Generative Artificial Intelligence (AI) stands at the forefront of technological innovation, revolutionizing the way machines understand and create content. This cutting-edge field employs advanced algorithms to enable computers not only to analyze and process data but also to generate novel and creative outputs autonomously. From generating realistic images and text to enhancing problem-solving capabilities, generative AI is unlocking new realms of possibilities, reshaping industries, and challenging the boundaries of what machines can achieve in the realm of creativity and ingenuity.
What is Generative AI?
How does Generative AI work?
Generative AI operates through the utilization of a machine learning (ML) model to grasp the intricacies and connections within a collection of human-generated material. Subsequently, it applies the assimilated patterns to produce novel content. The prevalent approach for instructing a generative AI model involves employing supervised learning.
In this method, the model receives a compilation of human-generated material alongside their associated labels. Consequently, it acquires the ability to produce content resembling human-generated material and labeled with identical classifications.
Benefits of Generative AI
Generative AI holds significance for various reasons. Several notable advantages of generative AI encompass:
Hence, generative AI stands poised to make a profound impact across diverse industries and applications, marking it as a crucial area of focus in AI research and development.
Applications of Generative AI
Here are the most popular generative AI applications:
- Language: Text serves as the cornerstone for numerous AI models geared towards generative tasks, representing a domain of high complexity. An illustrative instance of such models is the category of expansive language models (ELMs). These ELMs find utility across diverse domains, ranging from crafting essays, programming code, and language translation, to deciphering genetic sequences.
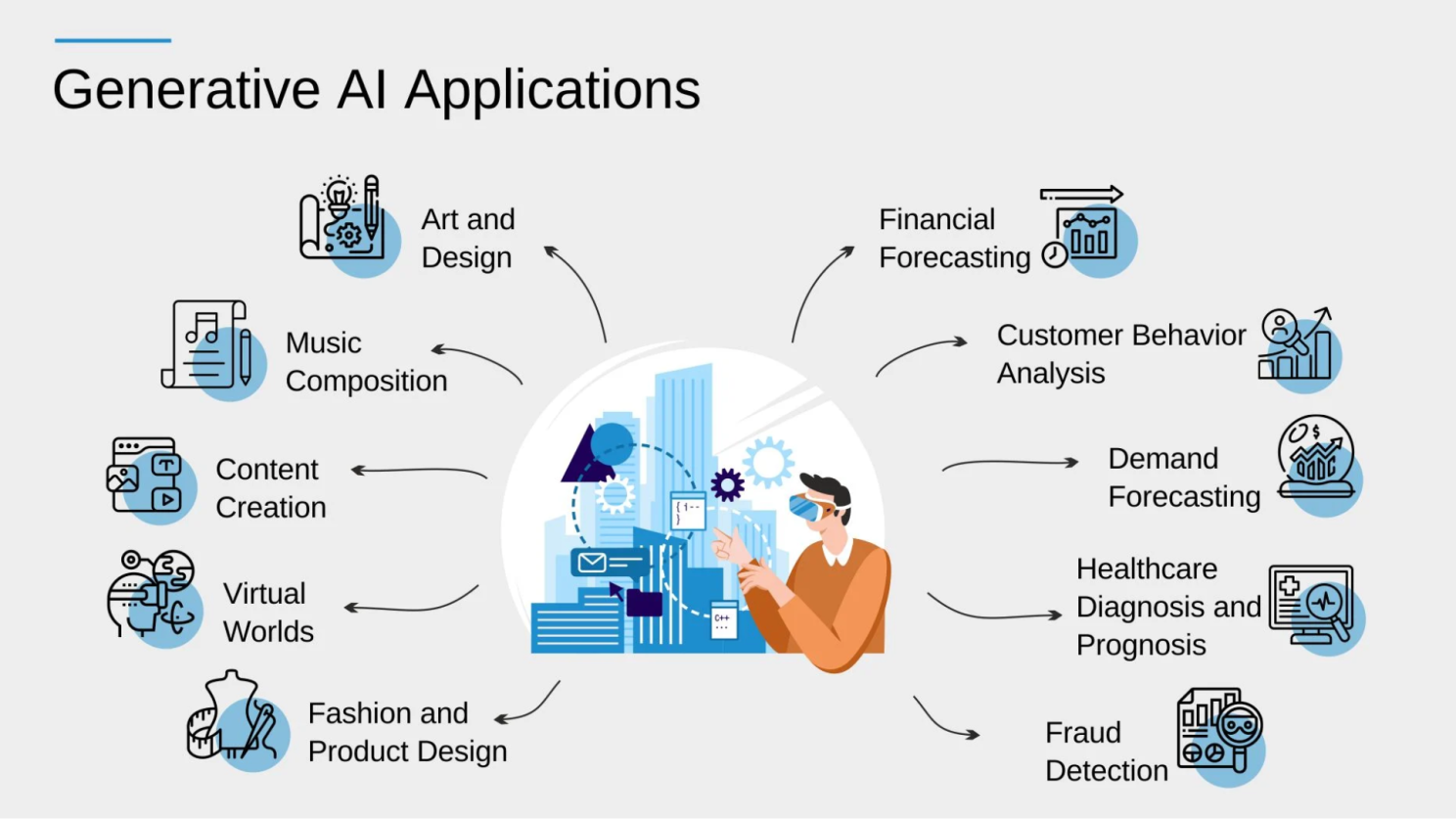
- Audio: The realms of music, audio, and speech constitute burgeoning frontiers in generative AI. Instances include models proficient in composing melodies and short audio snippets based on textual prompts, identifying objects within videos and providing corresponding sound effects, and even tailoring bespoke musical compositions.
- Visual: Within the domain of generative AI, image generation stands as one of the most prevalent applications. This domain encompasses the creation of three-dimensional imagery, virtual personas, video content, graphical representations, among others. Noteworthy is the flexibility in crafting images with varied aesthetic nuances, as well as the ability to manipulate and refine generated visual content. Generative AI facilitates the creation of graphical representations elucidating novel chemical compounds vital for drug discovery, crafting lifelike visuals for virtual or augmented reality experiences, generating three-dimensional assets for gaming environments, designing brand logos, refining and embellishing existing imagery, and beyond.
- Synthetic Data: Synthetic data holds profound significance in training AI models, especially when authentic data is scarce, constrained, or insufficient for addressing niche scenarios with optimal precision. The synthesis of data through generative models stands as a pivotal solution for circumventing the data-related hurdles encountered by many enterprises. This approach spans diverse modalities and use cases, made feasible through methodologies such as label-efficient learning. Generative AI models mitigate labeling expenses by either autonomously generating augmented training data or acquiring an internal data representation conducive to training AI models with diminished reliance on labeled data.
The influence of generative models reverberates across myriad domains, with their utility continually expanding. The examples provided merely scratch the surface of how generative AI catalyzes advancements and reshapes domains such as transportation, natural sciences, and entertainment.
- In the automotive sector, generative artificial intelligence (AI) is anticipated to play a pivotal role in crafting three-dimensional (3D) environments and models for simulations and the advancement of automobiles. Synthetic data is also finding application in the training of self-driving vehicles. The ability to assess the capabilities of autonomous vehicles on the road within a lifelike 3D environment not only enhances safety, efficiency, and flexibility but also mitigates risks and reduces operational overhead.
- The domain of natural sciences reaps substantial benefits from generative AI. Within the healthcare sector, generative models prove instrumental in medical research by generating novel protein sequences to facilitate drug discovery. Healthcare practitioners can further capitalize on task automation, encompassing activities like note-taking, medical coding, medical imaging analysis, and genomic study. Concurrently, in meteorology, generative models find utility in crafting simulations of Earth, contributing to precise weather predictions and the anticipation of natural disasters. These applications contribute to the creation of secure environments for the public and empower scientists to predict and prepare for unforeseen events.
- In the expansive realm of the entertainment industry, spanning video games, film, animation, world-building, and virtual reality, generative AI models serve as invaluable tools, streamlining the content creation process. Creators harness these models to complement their creativity and enhance their work without detection by widely used AI tools for assessing text originality.
Practical Applications of Generative AI
The realm of generative AI is advancing swiftly, with significant contributions to scientific exploration and technological advancement. Emerging applications span creative content generation, content enhancement, synthetic data generation, generative engineering, and generative design.
Present-day practical implementations include the following:
- Augmentation and creation of written content: Generating draft outputs of text in desired styles and lengths.
- Question answering and discovery: Facilitating users in locating answers based on input data and prompt information.
- Text tone manipulation: Adjusting language to soften or professionalize the text.
- Summarization: Providing concise versions of conversations, articles, emails, and web pages.
- Simplification: Breaking down titles, creating outlines, and extracting essential content.
- Content classification for specific use cases: Sorting content by sentiment, topic, etc.
- Enhancement of chatbot performance: Improving entity extraction, sentiment classification throughout conversations, and generating journey flows from general descriptions.
- Software coding: Assisting in code generation, translation, explanation, and verification.
Emerging use cases with long-term impacts encompass:
- Creating medical images depicting the future progression of diseases.
- Synthetic data generation to supplement limited data, address bias, protect data privacy, and simulate future scenarios.
- Applications suggest additional actions to users proactively and furnish them with relevant information.
- Modernization of legacy code.
Contributions of Generative AI in Business
Generative AI introduces novel and disruptive prospects for boosting revenue, curtailing costs, enhancing productivity, and refining risk management. Soon, it is poised to emerge as a competitive advantage and a key differentiator in the business landscape.
Gartner categorizes these opportunities into three main domains:
Revenue Opportunities:
Cost and Productivity Opportunities
Risk Opportunities:
Example of Industries using Generative AI
Generative AI is set to influence various industries, including pharmaceuticals, manufacturing, media, architecture, interior design, engineering, automotive, aerospace, defense, medical, electronics, and energy. It augments core processes within these sectors through the integration of AI models. Additionally, it impacts various organizational functions such as marketing, design, corporate communications, training, and software engineering, enhancing supporting processes across multiple domains. For instance:
- Pharmaceutical Industry
By 2025, we anticipate that over 30% of new drugs and materials will be systematically discovered using generative AI techniques, marking a significant advancement from the current zero utilization. The pharmaceutical sector stands to benefit from generative AI by reducing costs and expediting the drug discovery process.
-
Marketing
We project that by 2025, approximately 30% of outbound marketing messages from large organizations will be synthetically generated, a substantial increase from the less than 2% recorded in 2022. Text generators like GPT-3 are already proving their capability in creating marketing copy and personalized advertising.
-
Manufacturing, Automotive, Aerospace, and Defense Industries
Generative design is revolutionizing these sectors by creating optimized designs tailored to meet specific objectives and constraints, including performance, materials, and manufacturing methods. This acceleration in the design process offers engineers a multitude of potential solutions to explore.
Risks/Shortcomings of Generative AI
As a dynamic field, generative models are still in their early phases, leaving ample room for advancement in various aspects:
- Scale of Compute Infrastructure
Generative AI models, boasting billions of parameters, demand fast and efficient data pipelines for training. Maintaining and developing such models requires significant capital investment, technical expertise, and large-scale computing infrastructure. For example, diffusion models may necessitate millions or billions of images for training, requiring substantial computing power, with AI practitioners procuring and leveraging hundreds of GPUs to train their models.
- Sampling Speed
The scale of generative models can introduce latency in generating instances. This is particularly crucial for interactive use cases like chatbots, AI voice assistants, or customer service applications, where immediate and accurate responses are essential. As diffusion models gain popularity for their high-quality samples, the challenge of slow sampling speeds becomes more evident.
- Lack of High-Quality Data
Generative AI models often produce synthetic data for various use cases. However, not all the data generated globally is suitable for training AI models. Generative models demand high-quality, unbiased data to function effectively. Some domains lack sufficient data for model training; for instance, developing 3D assets is expensive and limited. Addressing these gaps requires substantial resources for evolution and maturity.
- Data Licences
Obtaining commercial licenses for existing datasets or building custom datasets for training generative models is a challenge faced by many organizations. This crucial process is vital for avoiding intellectual property infringement issues
Various companies, such as NVIDIA, Cohere, and Microsoft, are committed to supporting the ongoing growth and development of generative AI models. They offer services and tools that simplify the complexities of setting up and running these models at scale.
Future of Generative AI in Business
In the business realm, numerous individuals serve as content creators across various domains. The advent of Generative AI will profoundly transform their roles, whether it involves generating text, images, hardware designs, music, videos, or other forms of content. Consequently, workers will need to transition into the role of content editors, requiring a distinct skill set from that of content creation.
Simultaneously, the dynamics of workforce interaction with applications are evolving, as applications become more conversational, proactive, and interactive. This shift necessitates a redesigned user experience. In the short term, generative AI models will not only respond to natural language queries but also suggest ideas or solutions that users may not have explicitly requested. For instance, if you ask for a data-driven bar chart, the model might propose alternative graphics it deems relevant. In theory, this shift may enhance worker productivity, challenging conventional notions about the necessity for humans to exclusively lead in strategy development.
The overall impact on the workforce will vary significantly based on factors such as industry, location, enterprise size, and the range of offerings.
Wrapping Up!
In a nutshell, the transformative capabilities of generative AI hold immense promise across diverse industries, signaling a paradigm shift in how we approach problem-solving and innovation. As an integral focus of ongoing AI research and development, its potential applications are boundless, promising unprecedented advancements in fields ranging from healthcare to entertainment. Embracing and harnessing the power of generative AI is not just a technological leap but a key driver for shaping the future landscape of various sectors, paving the way for a more dynamic and efficient world.